Development of a Model to Prevent Drought of Lake Mead Water Reservoir
Authors
Chi Wa Wu, Shengying Zhao
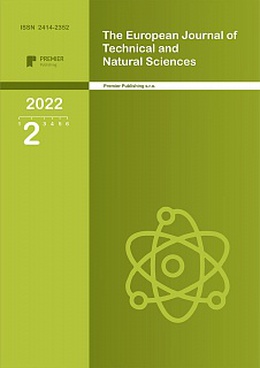
Share
Annotation
Climate change has been a prominent subject in recent decades as it silently alters our lives. Droughts have become more common, resulting in the decrease of the Lake Mead water reservoir in the United States. To keep track of the situation, we'll need to simulate the trend in elevation, surface area, and volume, which collectively define the water level. To combat the drought, we'll need to develop drought criteria for Lake Mead, as well as feasible programs and techniques for measuring the impact.
In this paper, we will finally develop a time series model to predict the water level and help people and government to prepare in advance for the drought period.
Authors
Chi Wa Wu, Shengying Zhao
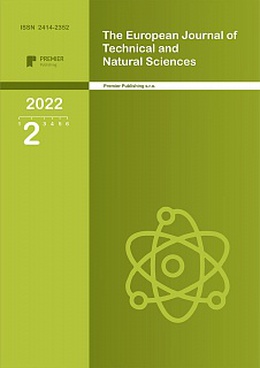
Share
References:
- Kwiatkowski, D., Phillips, P. C. B., Schmidt, P., & Shin, Y. (1992). Testing the null hypothesis of stationarity against the alternative of a unit root: How sure are we that economic time series have a unit root? Journal of Econometrics, 54(1-3), 159–178.
- Wei, W. W. (2006). Time series analysis. In The Oxford Handbook of Quantitative Methods in Psychology: Vol. 2.
- Keddy, P. A., & Reznicek, A. A. (1986). Great Lakes vegetation dynamics: the role of fluctuating water levels and buried seeds. Journal of Great Lakes Research, 12(1), 25-36.
- Brook, A. J., & Woodward, W. B. (1956). Some observations on the effects of water inflow and outflow on the plankton of small lakes. The journal of Animal Ecology, 22-35.
- Anderson, J. (2003). The environmental benefits of water recycling and reuse. Water Science and Technology: Water Supply, 3(4), 1-10.
- Angelakis, A. N., Bontoux, L., & Lazarova, V. (2003). Challenges and prospectives for water recycling and reuse in EU countries. Water Science and Technology: Water Supply, 3(4), 59-68.
- Sorjamaa, A., Hao, J., Reyhani, N., Ji, Y., & Lendasse, A. (2007). Methodology for long-term prediction of time series. Neurocomputing, 70(16-18), 2861-2869.
- Karakoyun, E. S., & Cibikdiken, A. O. (2018, May). Comparison of arima time series model and lstm deep learning algorithm for bitcoin price forecasting. In The 13th multidisciplinary academic conference in Prague (Vol. 2018, pp. 171-180).
- Hall, A. (1994). Testing for a unit root in time series with pretest data-based model selection. Journal of Business & Economic Statistics, 12(4), 461-470.
- Mitchell, V. G., Mein, R. G., & McMahon, T. A. (2001). Modelling the urban water cycle. Environmental Modelling & Software, 16(7), 615-629.
- Lucchetti, G. L., & Gray, G. A. (1988). Water reuse systems: a review of principal components. The Progressive Fish-Culturist, 50(1), 1-6.
- Barnett, T. P., & Pierce, D. W. (2008). When will Lake Mead go dry?. Water Resources Research, 44(3).
- Harbeck, G. E. (1958). Water-loss investigations: Lake Mead studies (Vol. 298). US Government Printing Office.
- Achhami, A., Kalra, A., & Ahmad, S. (2018, May). Dynamic simulation of Lake Mead water levels in response to climate change and varying demands. In World Environmental and Water Resources Congress 2018: Watershed Management, Irrigation and Drainage, and Water Resources Planning and Management (pp. 260-268). Reston, VA: American Society of Civil Engineers.