DEVELOPING A PREDICTIVE MODEL FOR EMOTIONAL AND BEHAVIORAL DISORDER AMONG CHILDREN
Authors
Yicen Tao
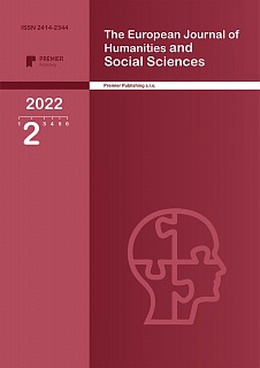
Share
Annotation
Emotional and behavioral disorder (EBD) has been an ongoing issue in the United States. According to CDC, about 17% children and teenagers are reported to have symptoms of EBD. Thus, it is imperative for groups like parents, guardians, doctors, and teachers to develop a more in-depth understanding of the causes that lead to EBD. The data in this study are the responses of 67,625 individuals to the survey National Survey of Children’s Health (NSCH). Mean value imputation, and min-max scaling are some of the pre-processing techniques that we used to prepare the data set for later model-building. Then we selected some independent variables including demographic information, and special therapy status, and used them to develop two predictive models, a logistic regression model and an artificial neural network, in order to predict the probability of developing EBD. Furthermore, we used AUC and ROC to evaluate the accuracy of the two models. Both predictive models have good performance. The AUC of the two predictive models are 0.93 and 0.94, respectively, and the ROC curve of the models are also good and similar. The results indicate that older children are more likely to have EBD, while the children who are receiving or have received special therapy for mental problems are less likely to develop EBD. This report concludes that offering quality health care to the children in need is an effective way to decrease their probability of developing EBD.
Keywords
Authors
Yicen Tao
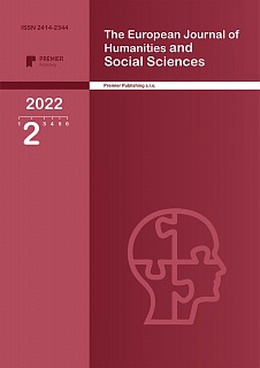
Share
References:
- CDC (2021). “Data and Statistics on Children's Mental Health.”, Centers for Disease Control and Prevention, 22 Mar. 2021, www.cdc.gov/childrensmentalhealth/data.html.
- Garcia-Arocena, D. (2015). Happy or sad: The chemistry behind depression. The Jackson Laboratory. https://www.jax.org/news-and-insights/jax-blog/2015/december/happy-or-sad-the-chemistry-behind-depression#.
- Garson, G.D. 1991. Interpreting neural network connection weights. Artificial Intelligence Expert. 6(4):46-51.
- Google. (2020 Aug. 11). Classification: ROC curve and Auc | machine Learning crash course. Google. https://developers.google.com/machine-learning/crash-course/classification/roc-and-auc.
- National Survey of Children's Health - Data Resource Center for Child and Adolescent Health, 2019, www.childhealthdata.org/learn-about-the-nsch/NSCH.
- Stefan Fritsch, Frauke Guenther and Marvin N. Wright (2019). neuralnet: Training of Neural Networks. R package version 1.44.2. https://CRAN.R-project.org/package=neuralnet
- Wang, Sijie, et al. "Exposure to Melamine cyanuric acid in adolescent mice caused emotional disorder and behavioral disorder." Ecotoxicology and Environmental Safety 211 (2021): 111938.