Empirical Study on Regulatory Sandbox Application Based on Simulation and Reinforcement Learning
Authors
Yuxuan Yang
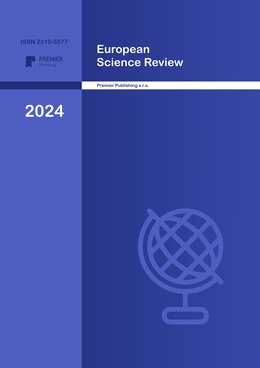
Share
Annotation
. This paper addresses the challenge of risk pricing in commercial banks amid increasing systemic risks influenced by global economic fluctuations, policy adjustments, and major global events. We introduce a novel framework combining digital twin technology and deep reinforcement learning to aid in more effective interest rate pricing decisions. By constructing a digital twin environment that simulates the operational conditions of commercial banks under various scenarios, and employing deep reinforcement learning models, the framework aims to devise optimal interest rate strategies that align with the banks' objectives. Our empirical analyses demonstrate the superiority of this AI-driven approach over traditional expert pricing methods, offering a robust decision support system for managing risk pricing in commercial banks.
Keywords
Authors
Yuxuan Yang
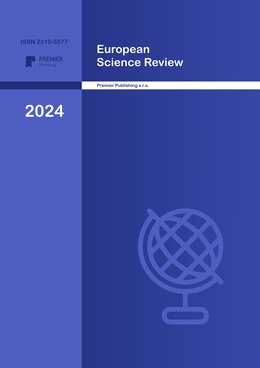
Share
References:
Collins, A., Sokolowski, J., & Banks, C. (2013). Applying reinforcement learning to an insurgency agent-based simulation. The Journal of Defense Modeling and simulation Applications Methodology Technology, 11(4), 353-364. https://doi.org/10.1177/1548512913501728
Sivamayil, K., Elakkiya, R., Aljafari, B., Nikolovski, S., Subramaniyaswamy, V., & Indragandhi, V. (2023). A systematic study on reinforcement learning based applications. Energies, 16(3), 1512. https://doi.org/10.3390/en16031512
Vokhidova, M.K., Abdullaeva, A.R. (2024). Directions of Trade Relations of Uzbekistan with the Countries of Central Asia. In: Sergi, B.S., Popkova, E.G., Ostrovskaya, A.A., Chursin, A.A., Ragulina, Y.V. (eds) Ecological Footprint of the Modern Economy and the Ways to Reduce It. Advances in Science, Technology & Innovation. Springer, Cham. https://doi.org/10.1007/978-3-031-49711-7_76
Mahmud, M., Kaiser, M., Hussain, A., & Vassanelli, S. (2018). applications of deep learning and reinforcement learning to biological data. Ieee Transactions on Neural Networks and learning Systems, 29(6), 2063-2079. https://doi.org/10.1109/tnnls.2018.2790388
Deeka, T., Deeka, B., & On-rit, S. (2021). A study of a competitive reinforcement learning approach for joint spatial division and multiplexing in massive mimo. Ecti Transactions on Electrical Engineering Electronics and Communications, 19(1), 83-93. https://doi.org/10.37936/ecti-eec.2021191.226832