IMPLICATIONS OF CREDIT APPROVAL
Authors
Hantong Li
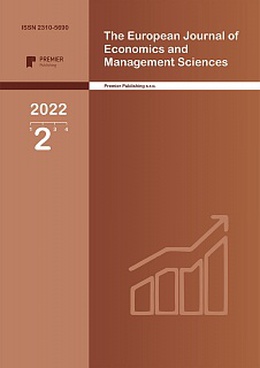
Share
Annotation
Since the advance of the information era, much research has been conducted to help applicants successfully apply for loans. While studies have examined financially related factors, such as income and socioeconomic status, few studies explore aspects outside the financial realm, such as gender and a few other factors. With the loan application data from the Dream Housing Finance Company, we used a logistic regression model to analyze the association between loan approval and other variables. Then, to examine the high correlation impact on the model result, a lasso logistic regression is also fitted, and the out-of-sample performance is compared. We have found that among all the factors, marriage status, the presence of credit history, and geographical locations have significant effects on the application results. In the end, the data is segmented according to male and female groups to investigate the difference in feature importance across gender. The result indicates that female applicant is additionally judged by their income and their co-applicant income, which suggests that women are still, or perceived as, financially unstable in modern society.
Keywords
Authors
Hantong Li
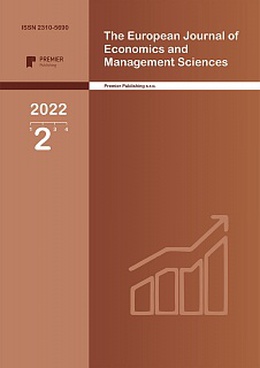
Share
References:
[1]: Kaggle. “Loan Data Set.” kaggle.com. https://www.kaggle.com/burak3ergun/loan-data-set.
[2]: Arun, Kumar, Garg Ishan, and Kaur Sanmeet. "Loan approval prediction based on machine learning approach." IOSR J. Comput. Eng 18.3 (2016): 18-21.
[3]: Siles, Marcelo, Steven D. Hanson, and Lindon J. Robison. "Socio‐economics and the probability of loan approval." Applied Economic Perspectives and Policy 16.3 (1994): 363-372.
[4]: Investopedia, and Thomas Brock. “Credit History Definition.” Investopedia, 2019. https://www.investopedia.com/terms/c/credit-history.asp.
[5]: Insurance Information Institute. “Credit Score vs Credit History | III.” www.iii.org. Accessed July 17, 2021. https://www.iii.org/article/what-difference-between-my-credit-score-and-my-credit-history.
[6]: Tatham, Matt. “How Lenders View Your Credit.” Experian.com, September 6, 2018. https://www.experian.com/blogs/ask-experian/how-lenders-view-your-credit/.
[7]: Google. Classification: ROC Curve and AUC | Machine Learning Crash Course. Accessed November 25, 2021. https://developers.google.com/machine-learning/crash-course/classification/roc-and-auc.
[8]: Kozma, Laszlo. "k Nearest Neighbors algorithm (kNN)." Helsinki University of Technology (2008).