Predicting Breast Cancer using Artificial Neural Network and Logistic Regression
Authors
Cheng Annika
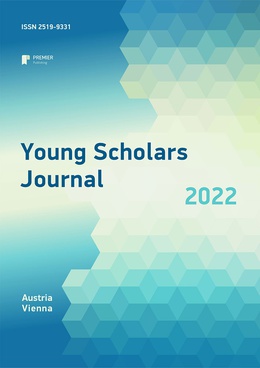
Share
Annotation
Objective: This study aims to build a predictive model for breast cancer using artificial neural network and compare its performance to logistic regression model.
Methods: Wisconsin Diagnostic Breast Cancer (WDBC) data was used in this study. Features were computed from a digitized image of a fine needle aspirate (FNA) of a breast mass. They described characteristics of the cell nuclei present in the image.
All the participants who were eligible were randomly assigned into 2 groups: training sample and testing sample. Two models were built using training sample: artificial neural network and logistic regression. We used these two models to predict the risk of breast cancer in the testing sample. Receiver operating characteristic (ROC) were calculated and compared for these two models for their discrimination capability and a curve using predicted probability versus observed probability were plotted to demonstrate the calibration measure for these two models.
Results: A total of 569 patients were included in this analysis, 357 (62.74%) benign, 212 (37.26%) malignant breast cancer patients.
According to the logistic regression, number of concave portions of the contour and texture (standard deviation of gray-scale values) were at important predictors for malignant breast cancer.
According to this neural network, the top 5 most important predictors were worst area, mean of severity of concave portions of the contour, worst of severity of concave portions of the contour, worst of symmetry, worst of compactness.
For training sample, the ROC was 1.0 for the Logistic regression and 1.0 for the artificial neural network. Artificial neural network performed better clearly. While in testing sample, the ROC was 0.92 for the Logistic regression and 0.99 for the artificial neural network. Artificial neural network had better performance.
As to calibration measure, predictions made by the neural network are (in general) less concentrated around the 45-degree line (a perfect alignment with the line would indicate an ideal perfect calibration) than those made by the Logistic model.
Conclusions: In this study, we identified several important predictors for breast cancer e.g., number of concave portions of the contour, worst of symmetry, worst of compactness. This provided important information for providers and patients for timely accurate diagnosis. We built a predictive model using artificial neural network as well as logistic regression to provide a tool for timely accurate diagnosis. When compared to artificial neural network model, logistic regression had a worse discriminating capability and a better calibration between predicted probability and observed probability.
Authors
Cheng Annika
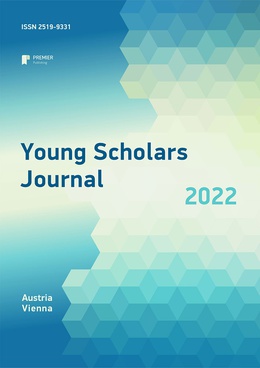
Share
References:
[1] U.S. Cancer Statistics Working Group. United States Cancer Statistics: 1999–2014 Incidence and Mortality Web-based Report. Atlanta (GA): Department of Health and Human Services, Centers for Disease Control and Prevention, and National Cancer Institute; 2017
[2] Antoniou A, Pharoah PD, Narod S, et al. Average risks of breast and ovarian cancer associated with BRCA1 or BRCA2 mutations detected in case series unselected for family history: A combined analysis of 22 studies. American Journal of Human Genetics 2003; 72(5):1117–1130.
[3] Chen S, Parmigiani G. Meta-analysis of BRCA1 and BRCA2 penetrance. Journal of Clinical Oncology 2007; 25(11):1329–1333
[4] CDC. Risk Factors for Breast Cancer in Young Women. https://www.cdc.gov/cancer/breast/young_women/risk_factors.htm
[5] K. P. Bennett and O. L. Mangasarian: "Robust Linear Programming Discrimination of Two Linearly Inseparable Sets", Optimization Methods and Software 1, 1992, 23-34